Over 2,500 years’ worth of human learning and research toppled by a machine: In an intellectual world that considers mastery of its domain a quest of ultimate scholarship and cultivation, a computer overtook everyone. And then, a revised version relearned its skill in just a matter of days.
Welcome to the world of the strategy board game Go, which originated in China before 500 BC and is played by tens of millions of people. The Go community was deeply shaken by staunch wins of DeepMind’s AlphaGo program over top professionals including Ke Jie, the highest ranked player in the world. After his defeat, Ke Jie commented on Social Media: “After humanity spent thousands of years improving our tactics, computers tell us that humans are completely wrong, … I would go as far as to say not a single human has touched the edge of the truth of Go”[1].
But soon Go players looking for a competitive edge embraced AlphaGo and its successors as a fresh set of tools, and are now studying its unique non-human playing style to find better moves. Indeed, machine learning is already being applied almost everywhere where data is being generated, and can be credited with creating value with insights, predictions, decision making and automation across a plethora of applications – as well as a considerable amount of hype.[2] What does the story of machine learning overtaking the world of Go tell us about learning from machine learning in the realm of business or commercial enterprise?
Go enthusiasts liken the game to an art form, and indeed it was historically one of the constituents of the four arts of the Chinese scholar. The board presents a blank canvas upon which a beautiful interplay between two life forces is enacted. In contrast, we may say that a Go playing machine cannot appreciate the game; it simply knows ‘how’, but not ‘why’ or even ‘what’ it is playing. Nevertheless, DeepMind’s AlphaGo, and its Artificial Neural Networks (ANNs)[3], demonstrate that the state of the art is capable of identifying pertinent patterns in a virtually limitless space that humans struggle to visualise.
To make the most of machine learning, we should look at what we are doing and recognise what we do best… as humans that is.
Machine learning requires data. Wherever we collect data, it is at best a shadow of reality. A projection of snapshots captured through whatever devices and sensors we are using. In the same way that we can’t fully reconstruct reality from even the highest resolution recording technology, we can only feed machines with a bare subset of reality, stripped of context and meaning.
This helps to explain why Chess and Go are among the first human endeavours seeing breakthroughs, because their reality is constrained to a handful of fundamental rules and there is nothing to digitise but the plainly visible positions of the pieces on the board.
In real-life applications of machine learning, the distance between the manifestation of our digital data and everything that is happening in reality is considerably greater. Besides, we know that human or measurement errors and statistical noise are always part of the mix.
Real-life applications
Take, for example, an investigative study for predicting the progression of diabetes for a group of patients diagnosed with the disease. The dataset was collected for an academic study and is based on just 10 demographic and clinical measurements [4].
As we might expect, because the data does not incorporate information about genetic disposition, lifestyle, diet or comorbidities, we found that training a global predictive model on the data did not yield useful predictions about disease progression for all patients. This is not down to a lack of machine learning sophistication – the data simply does not contain sufficient discerning information. However, because humans are well attached to reality, and machines are not, we are best placed to apply our domain knowledge to recognise this. Secondly, unlike machine learning algorithms, as we aren’t restricted to a pre-programmed remit, we have the freedom to think outside the box.
On re-examining the accuracy of the predicted disease progression across the cohort, we came up with the idea that some conditional cases might possibly be more predictable than others. Returning to our machine learning toolkit, we applied an unsupervised learning algorithm to map out the patient cohort and discover that there are indeed sub-groups that exhibit superior prediction accuracy. The visualisation below renders them in bolder colours: blue for lowest, yellow for intermediate and red for high disease progression together with a higher predictive certainty. The fainter and paler areas symbolise sub-groups of lower prediction accuracy.
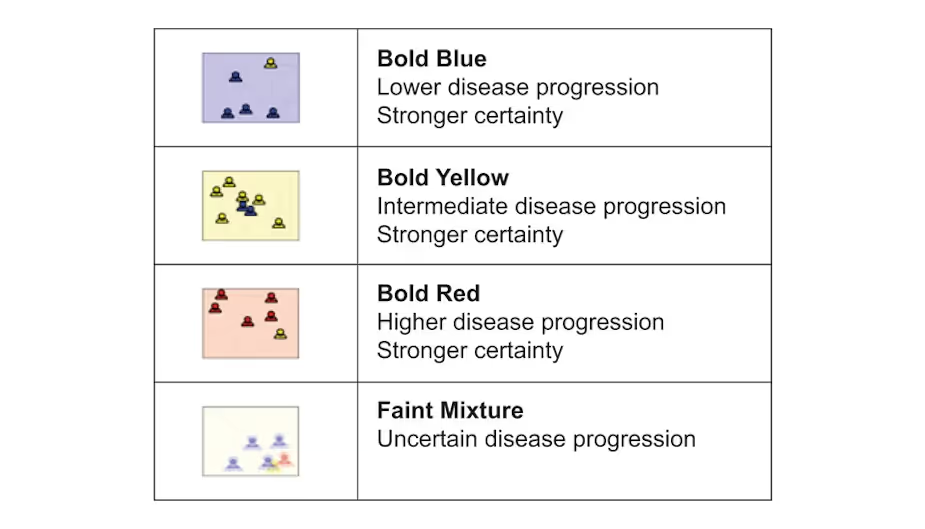
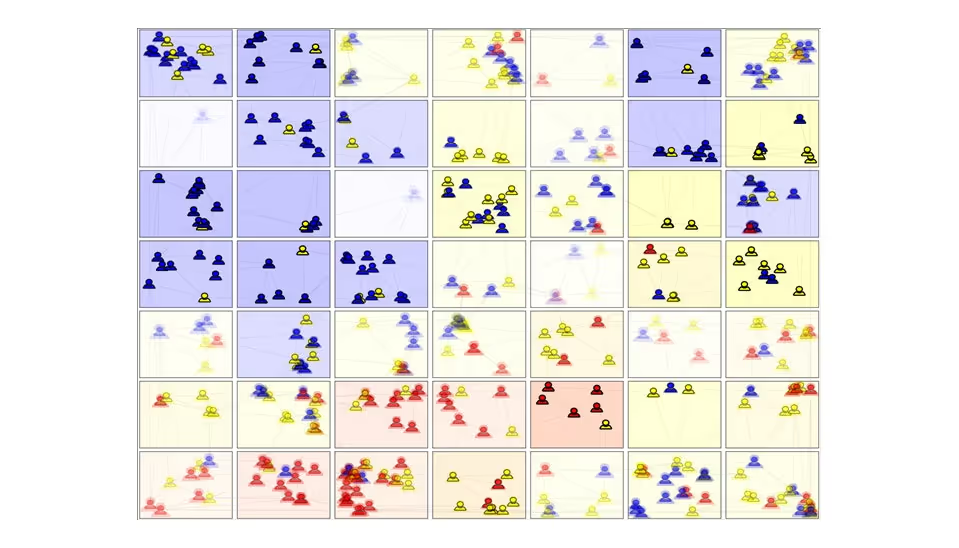
To tie this back to real-life applications, this partitioning of the cohort is analogous to the practice of ‘stratified healthcare’ or ‘precision medicine’, where the treatment prescribed to patients is not a solitary choice but selected for each individual qualified by a clinical assessment. A clinician could use the bolder areas of the map to advise those patients whether they are presently safely managing their diabetes or are at medium or high risk and require intervention. Patients in fainter areas may be advised that more information is required from them, which could include tracking diet, exercise or other activities alongside further clinical measurements.
While this small investigative study doesn’t represent a fully-fledged clinical project, we can take note of a few nuggets of insight. Machine learning is tremendously useful, capable of resolving patterns and relationships beyond the reach of human perception. It is, however, locked into its digital domain, working on an imperfect reflection of reality. Only we have the ability to bridge the gap between reality and the digital realm by thinking outside the box to refine our analysis and by applying domain knowledge to interpret the significance and application of the results. Having undertaken this study, we realise that we have improved our own understanding of reality and our analysis of it. It makes sense that not only is human intelligence a key component of machine learning, but that machine learning firmly reciprocates our own learning.
Lee Sedol, acclaimed as the Roger Federer of Go, has said that “robots will never understand the beauty of the game the same way that we humans do” [5]. After all, AlphaGo does not really ‘play’ Go, or exhibit any passion for the game. So, if we are teaming up with our new cool-headed but keen learning companion of machine learning, then by exercising our own capacity to experiment and discover, we become better equipped to engineer applications and solutions that work well in everyday life.
References
01. E. Dou and O. Geng, “Humans Mourn Loss After Google Is Unmasked as China’s Go Master,” The Wall Street Journal, April 2019.
03. S. Shakespeare, “AI – The Blinking Red Warning Light,” TTP, December 2018
05. M. L. Roux and P. Mollard, “Game over? New AI challenge to human smarts,” Phys.org, March 2016.